Understanding the Importance of R-Squared in Statistical Analysis
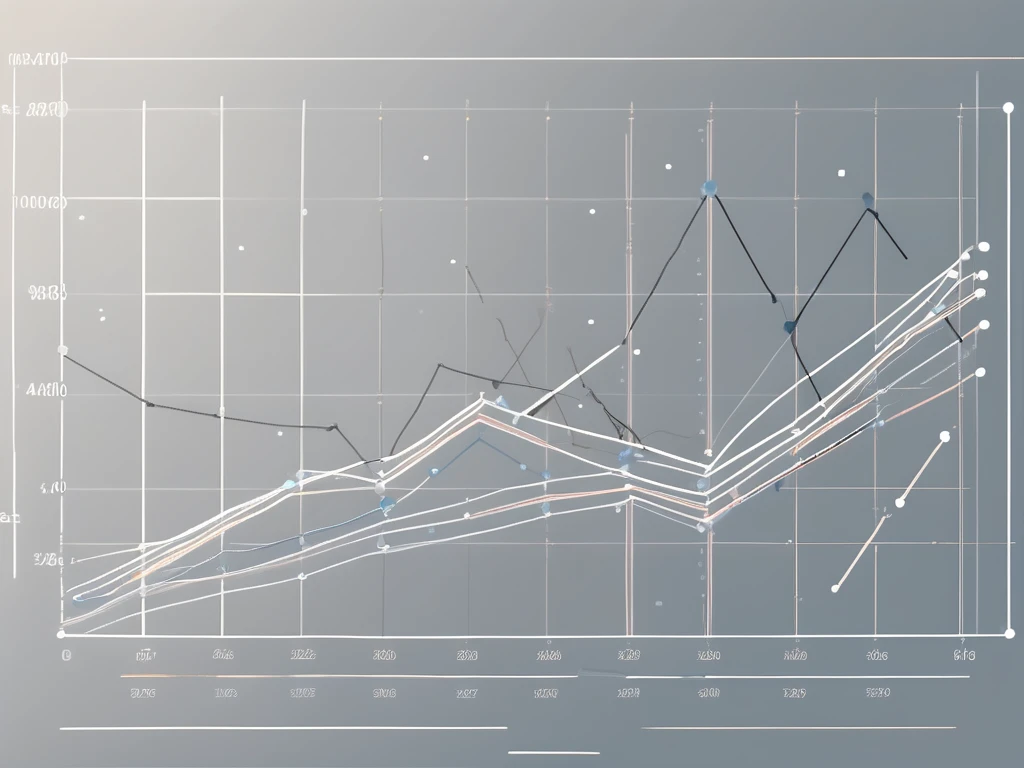
As a statistical analyst, I cannot stress enough the importance of understanding and utilizing R-squared in statistical analysis. R-squared is a vital metric that provides insights into the goodness of fit of a regression model. In this article, I will guide you through the concept of R-squared, its role in statistical analysis, its interpretation, limitations, and how it can be improved with adjusted R-squared. So, if you’re ready to up your statistical analysis game, let’s dive right in!
Defining R-Squared: A Brief Overview
Before we delve into the nitty-gritty, let’s get a solid understanding of what exactly R-squared is. R-squared, also known as the coefficient of determination, measures the proportion of the variance in the dependent variable that can be explained by the independent variables in a regression model. In simpler terms, it quantifies how well the regression model fits the observed data points.
The Concept of R-Squared
The concept behind R-squared is to determine the proportion of the total variation in the dependent variable that can be attributed to the independent variables. A value of 1 indicates that the regression model perfectly predicts the dependent variable, while a value of 0 indicates that the model doesn’t explain any of the variability in the dependent variable. It’s crucial to note that R-squared only reveals the goodness of fit and not the causality between variables.
Let’s consider an example to illustrate the concept of R-squared. Imagine we have a dataset containing information about the average temperature and ice cream sales in a particular city over several months. We want to build a regression model to predict ice cream sales based on the average temperature. By calculating the R-squared value, we can determine how much of the variability in ice cream sales can be explained by changes in temperature.
If the R-squared value is close to 1, it means that the regression model is highly accurate in predicting ice cream sales based on temperature. On the other hand, if the R-squared value is close to 0, it suggests that temperature has little to no impact on ice cream sales, and other factors may be influencing the sales.
The Mathematical Formula of R-Squared
To calculate R-squared, we use a mathematical formula based on the sum of squared errors (SSE) and the total sum of squares (SST). R-squared is calculated as 1 minus the ratio of SSE to SST. A higher R-squared value implies a better-fitting regression model, as it indicates that a larger proportion of the variance in the dependent variable is explained by the independent variables.
Let’s break down the formula further. The sum of squared errors (SSE) represents the sum of the squared differences between the observed values and the predicted values from the regression model. It measures the overall discrepancy between the actual data points and the model’s predictions.
On the other hand, the total sum of squares (SST) represents the sum of the squared differences between the observed values and the mean of the dependent variable. It measures the total variability in the dependent variable, regardless of the regression model.
By dividing SSE by SST and subtracting the result from 1, we obtain the R-squared value. This value ranges from 0 to 1, with 1 indicating a perfect fit and 0 indicating no fit at all.
It’s important to note that R-squared has its limitations. It can be influenced by outliers, the number of independent variables, and the presence of multicollinearity. Additionally, R-squared alone cannot determine the validity of a regression model. Therefore, it’s crucial to consider other statistical measures and conduct a thorough analysis before drawing conclusions based solely on R-squared.
The Role of R-Squared in Statistical Analysis
Now that we have a solid understanding of R-squared, let’s explore its role in statistical analysis.
Statistical analysis is a fundamental component of many fields, including economics, psychology, and biology. It involves the collection, organization, and interpretation of data to uncover patterns, relationships, and trends. One of the key tools in statistical analysis is regression analysis, which allows researchers to model the relationship between a dependent variable and one or more independent variables.
R-Squared as a Coefficient of Determination
R-squared is widely used as a coefficient of determination to evaluate the predictive power of a regression model. It helps researchers determine how well the chosen independent variables explain the variability in the dependent variable. In other words, R-squared measures the proportion of the variance in the dependent variable that can be explained by the independent variables included in the model.
For example, let’s say a researcher is interested in studying the relationship between a person’s age and their income. They collect data from a sample of individuals and use regression analysis to build a model that predicts income based on age. The R-squared value of this model indicates how much of the variation in income can be attributed to age. A higher R-squared value suggests that the model provides a better fit to the observed data, indicating that age is a significant predictor of income.
Understanding the Goodness of Fit
R-squared plays a crucial role in assessing the goodness of fit of a regression model. It helps analysts determine the appropriateness of the model in explaining the observed data variability. By comparing R-squared values across different models, you can identify the model that best fits the data and provides the most reliable predictions.
However, it is important to note that R-squared alone should not be the sole criterion for evaluating a model. While a high R-squared value indicates a good fit, it does not guarantee that the model is accurate or reliable. Other factors, such as the significance of the independent variables, the presence of multicollinearity, and the assumptions of the regression model, should also be considered when assessing the overall quality of the model.
In addition, it is worth mentioning that R-squared can be misleading in certain situations. For example, if a researcher includes irrelevant or redundant independent variables in the model, the R-squared value may be artificially inflated. This highlights the importance of careful variable selection and model specification in regression analysis.
In conclusion, R-squared is a valuable tool in statistical analysis, particularly in regression analysis. It helps researchers assess the predictive power of a model and determine the goodness of fit. However, it should be used in conjunction with other evaluation criteria to ensure the accuracy and reliability of the model.
Interpreting R-Squared Values
Now that we’ve established the role of R-squared, let’s discuss how to interpret its values.
What Does a High R-Squared Value Mean?
A high R-squared value, typically above 0.7 or 70%, indicates that a significant proportion of the variance in the dependent variable is explained by the independent variables. This suggests that the regression model is a good fit for the observed data and can reliably predict the dependent variable.
What Does a Low R-Squared Value Indicate?
On the other hand, a low R-squared value, below 0.3 or 30%, signifies that the regression model has little or no explanatory power. The independent variables in the model fail to explain a substantial portion of the variability in the dependent variable. In such cases, it’s essential to revisit the model and consider other variables that might better explain the observed data.
Limitations of R-Squared in Statistical Analysis
While R-squared is a valuable metric, it’s vital to acknowledge its limitations to avoid misinterpretations and derive accurate conclusions from your analysis.
Misinterpretations of R-Squared
R-squared should not be used to determine causality between variables. It only provides information about the fit of the regression model, not the underlying relationships between variables. Additionally, a high R-squared does not necessarily imply that the model is useful in predicting future outcomes. Always exercise caution and consider other statistical measures before drawing conclusions.
Situations Where R-Squared May Not Be Useful
R-squared may not be suitable when dealing with non-linear relationships between variables. In such cases, alternative metrics like adjusted R-squared or other model evaluation techniques should be employed. Also, R-squared can be sensitive to outliers, so it’s important to identify and handle them appropriately before relying solely on R-squared values for analysis.
Improving Model Accuracy with Adjusted R-Squared
Adjusted R-squared comes to the rescue when R-squared is inadequate in certain scenarios. Let’s explore how it can enhance model accuracy.
The Difference Between R-Squared and Adjusted R-Squared
Adjusted R-squared takes into account both the goodness of fit and the number of independent variables used in the regression model. Unlike R-squared, adjusted R-squared adjusts for the number of predictors, rewarding models that include fewer variables while maintaining comparable explanatory power. It provides a more accurate measure of how well the model generalizes new data.
When to Use Adjusted R-Squared
Adjusted R-squared is particularly useful when comparing models with a different number of independent variables. It helps identify the model that strikes the right balance between accuracy and simplicity. By considering adjusted R-squared, you can select a model that avoids overfitting while still explaining a significant portion of the dependent variable’s variance.
As an expert in statistical analysis, I’ve witnessed the transformative power of R-squared in various research projects. It enables researchers to gauge the strength of their regression models, make informed decisions, and derive meaningful insights. However, always remember to interpret R-squared values in conjunction with other statistical measures to obtain a comprehensive understanding of your data.
Frequently Asked Questions:
What is R-squared?
R-squared, or the coefficient of determination, measures the proportion of the variance in the dependent variable that can be explained by the independent variables in a regression model.
How do you interpret R-squared values?
A high R-squared indicates that a significant proportion of the variance in the dependent variable is explained by the independent variables, while a low R-squared suggests a weak relationship between the variables.
Can R-squared determine causality?
No, R-squared should not be used to establish causality between variables. It solely reflects the goodness of fit of the regression model, not the underlying relationships.
When should I use adjusted R-squared?
Adjusted R-squared is beneficial when comparing models with different numbers of independent variables. It penalizes models with excessive complexity and favors those that demonstrate good predictive power while using fewer variables.
Now armed with a solid understanding of R-squared, let your data analysis journey unfold with confidence and precision. Remember, numbers and statistics hold the key to unlocking valuable insights, and R-squared is an invaluable tool in your statistical arsenal.
As you harness the power of R-squared to unlock insights within your data, why not take your analytical prowess to the next level with Morpher? Morpher.com is not just a trading platform; it’s a revolution in the world of investing, utilizing blockchain technology to offer zero fees, infinite liquidity, and a unique trading experience across diverse asset classes. Whether you’re interested in stocks, cryptocurrencies, or even niche markets like NFTs, Morpher empowers you with fractional investing, short selling without interest fees, and up to 10x leverage. Embrace the future of trading with the safety and control of a non-custodial Morpher Wallet. Sign Up and Get Your Free Sign Up Bonus today to transform your trading experience with Morpher.

Disclaimer: All investments involve risk, and the past performance of a security, industry, sector, market, financial product, trading strategy, or individual’s trading does not guarantee future results or returns. Investors are fully responsible for any investment decisions they make. Such decisions should be based solely on an evaluation of their financial circumstances, investment objectives, risk tolerance, and liquidity needs. This post does not constitute investment advice.
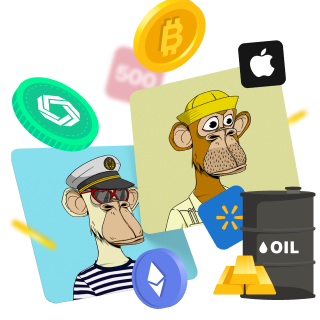
Painless trading for everyone
Hundreds of markets all in one place - Apple, Bitcoin, Gold, Watches, NFTs, Sneakers and so much more.
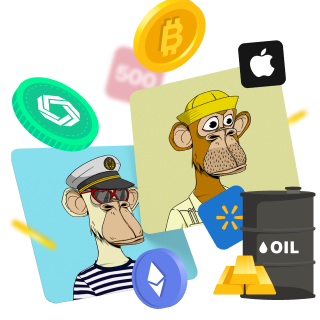
Painless trading for everyone
Hundreds of markets all in one place - Apple, Bitcoin, Gold, Watches, NFTs, Sneakers and so much more.